spk.so: Your Scientific Papers as AI-Generated Audio Dialogues
Prepend this domain to any scientific PDF to have it played back to you as an audio AI conversation, eg. spk.so/https://arxiv.org/pdf/1901.11004
The page will redirect to play the audio shortly. This is probably because you have not interacted with this site before and the browser does not allow auto play of audio. Alternatively, you can press the play button above now to play the audio.

Welcome to our discussion on the Stanford MLab's contribution to the SemEval 2022 Task 7, which focused on identifying plausible clarifications of implicit and underspecified phrases in instructional texts. This fascinating study reveals the challenges of understanding context and selecting the most fitting words or phrases in complex sentences. Now, let's dive into the main concepts and findings of the paper.
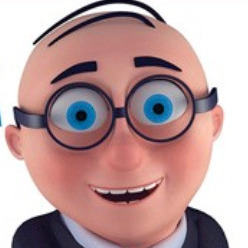
Absolutely! This research is significant because it tackles the practical issue of improving clarity in instructional texts, such as those found on platforms like wikiHow. The goal was to identify which phrases could best replace gaps in instructions, ensuring readers can easily follow along without confusion. What I find interesting is the diverse range of methods they explored, from tree-based techniques to transformer models like BERT and ELECTRA.

Yeah, the dataset they utilized included around 2.7 million sentences with revision histories, which provides a rich context for evaluating language models. Their task was twofold: first, to classify candidate phrases as plausible or implausible, and second, to score the plausibility on a scale from one to five. It’s a comprehensive approach that highlights the model’s understanding of language beyond surface-level recognition.
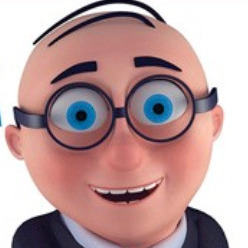
Exactly! They built several models, starting with traditional GloVe embeddings and incorporating advanced transformer architectures. The results they achieved with BERT, particularly the ELECTRA variant, suggest that these transformer-based methods significantly outperformed more classical approaches. I mean, can you talk about some of the specific results they found in this research?

Sure! In terms of accuracy, the ELECTRA model topped their results with a score of 0.465 on the dev set, while BERT scored slightly lower at 0.447 accuracy. Interestingly, the GloVe-based methods achieved much lower performance, underscoring the power of context-aware models like BERT. The study also highlighted that while the Infilling Language Model (ILM) approach seemed promising initially, it ultimately underperformed in comparison to the robust BERT-like models.
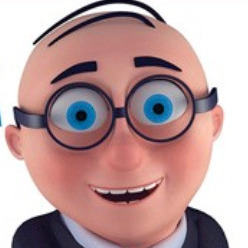
It's great to see how the models are evolving and performing. The findings raise questions about how well these models can generalize across different types of instructional text. How do you think the implications of this research could manifest in real-world applications?

Great point! The implications are vast. If these models can accurately predict plausible clarifications, they could be integrated into educational tools, making instructional content much more accessible and user-friendly. Also, companies that rely on instructional texts, like DIY websites or cooking platforms, could utilize these advancements to automatically suggest better phrasing and clarity in their guides.
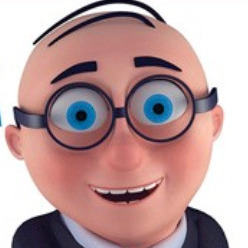
That would be super helpful! Additionally, the study emphasizes the importance of understanding context when it comes to language prediction. Given the rise of conversational AI, improving models to understand contextually nuanced language is essential for enhancing user interactions.

Absolutely. And looking ahead, the authors suggest that using even larger pretrained models like SpanBERT could enhance results, especially with multi-token span predictions. The direction of future research seems promising, focusing on refining these tools further to tackle more complex linguistic challenges. What do you think will be the next steps for research in this domain?
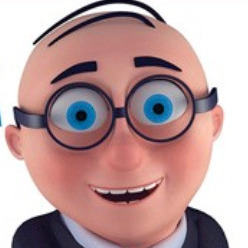
I imagine that we’ll see more frameworks that combine different models for better performance, especially in tasks involving longer phrases or complex sentences. Also, the specificity of tasks in natural language processing is becoming more pronounced, so researchers might tailor models even more closely to specific types of text or user interactions.

Definitely. It’s an exciting time for AI and NLP research! We encourage listeners to think about the next time they’re reading an instructional text, and how AI technologies could make those texts clearer and more effective. Thank you all for joining our engaging discussion today!